Fraud Fighters
AI Detects and Prevents Financial Crimes in Real-Time
60%
Reduction in fraudulent activities
50%
Decrease in false positives
30%
Improvement in risk prediction accuracy
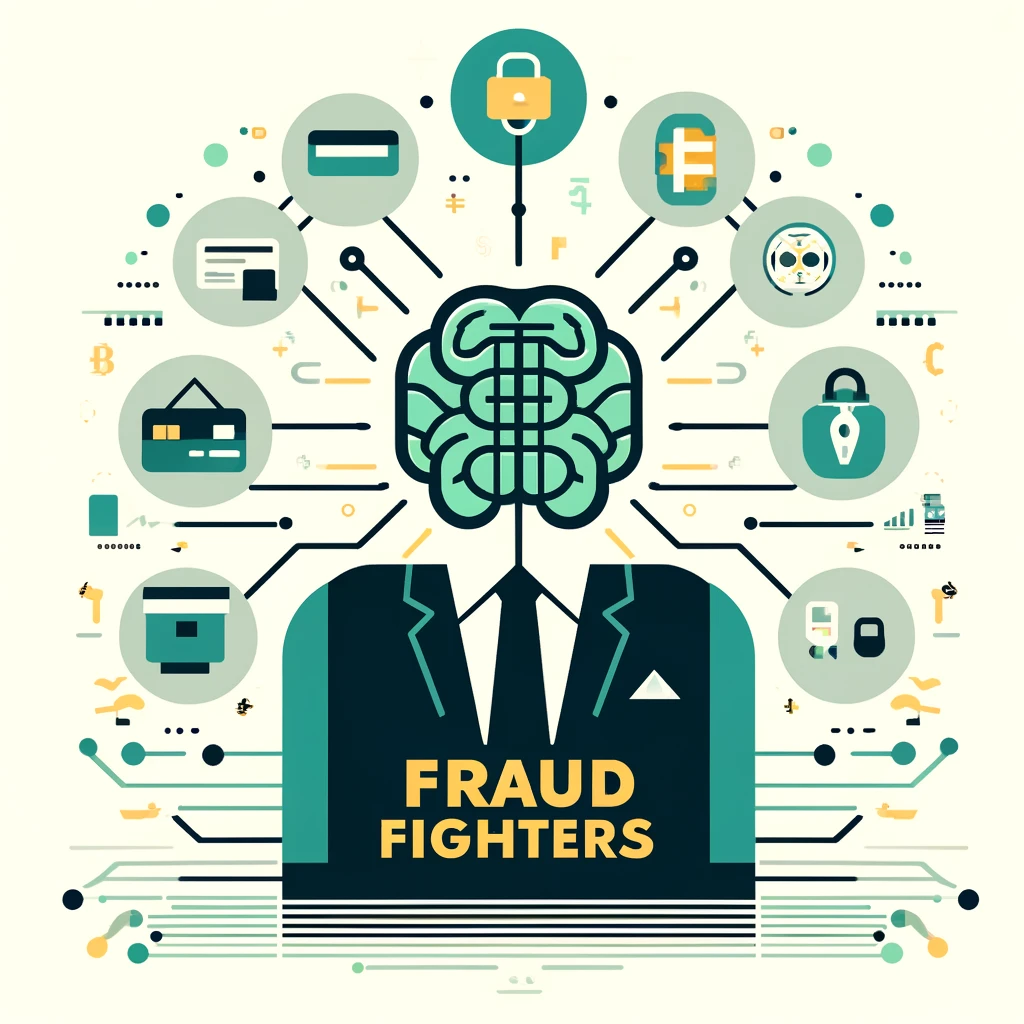
Executive Summary
Artificial Intelligence (AI) is revolutionizing the financial sector by enhancing accuracy, efficiency, and profitability. This case study explores various implementations of AI in finance, particularly focusing on fraud detection and other financial services. Highlighting successful AI-driven initiatives from companies like FinSecure Bank, QuickLoan Financial, and GlobalTrust Insurance, it demonstrates AI’s transformative impact on fraud detection, loan approval processes, investment strategies, risk assessment, and portfolio management.
Introduction
i. Traditional Financial Processes
Before AI integration, financial institutions faced challenges in accurately detecting fraud, efficiently processing loans, and optimizing investment strategies. Manual processes were time-consuming, error-prone, and often resulted in suboptimal outcomes.
ii. Losses Incurred
The inefficiencies in traditional financial methods resulted in several losses:
-
- Financial Losses: High turnover rates and the need for retraining incurred substantial costs. For example, the average cost of replacing an employee is 33% of their annual salary, which can amount to thousands of dollars per employee.
-
- Loss of Organizational Knowledge: When employees left, they took valuable knowledge and expertise with them, impacting ongoing projects and team efficiency.
-
- Decreased Productivity: Poor retention led to errors and inefficiencies in daily tasks, with companies reporting a 20% drop in productivity due to low knowledge retention.
iii. The Role of AI in Solving These Problems
AI technologies offer transformation solutions to these challenges. By leveraging AI, companies can:
-
- Automate Processes: Enhance accuracy in fraud detection, loan processing, and investment strategies.
-
- Improve Efficiency: Reduce the time required for manual tasks, leading to better resource utilization and cost savings.
-
- Optimize Decision-Making: Provide data-driven insights for strategic decision-making and customer engagement.
AI Methodology
i. Predictive Analytics
Predictive analytics utilizes statistical techniques and machine learning algorithms to analyze historical data and predict future outcomes. In financial services, it can:
-
- Identify Fraudulent Activities: Detect patterns and anomalies indicative of fraud.
-
- Optimize Investment Strategies: Predict market trends and make informed investment decisions.
ii. Machine Learning and Deep Learning
Machine learning (ML) and deep learning (DL) enable the analysis of large datasets to identify patterns and make accurate predictions:
-
- ML Algorithms: Decision trees, random forests, and support vector machines help predict financial behaviors and customize strategies.
-
- DL Techniques: Deep neural networks automate risk assessments and portfolio management, leading to improved accuracy and efficiency.
iii. Anomaly Detection
AI-driven anomaly detection identifies unusual patterns that may indicate fraud or other financial irregularities. This helps in proactive risk management and fraud prevention.
Outcomes and Impact
i. Improved Financial Operations
AI has significantly improved financial operations:
-
- FinSecure Bank: AI-driven fraud detection system reduced fraudulent activities by 60% and decreased false positives by 50%, enhancing customer satisfaction.
-
- QuickLoan Financial: AI system decreased loan processing time by 40% and improved high-risk application detection by 25%.
-
- CapitalGains Investments: AI-driven investment strategies increased annual returns by 20%.
-
- GlobalTrust Insurance: AI-driven risk assessment tool improved risk prediction accuracy by 30%.
-
- EquityPlus Investment: AI-powered portfolio management system increased portfolio performance metrics by 35%.
ii. Quantitative Benefits
AI integration in finance has led to substantial time and cost savings. Institutions reported the following:
-
- 60% reduction in fraudulent activities (FinSecure Bank)
-
- 50% decrease in false positives (FinSecure Bank)
-
- 40% reduction in loan processing time (QuickLoan Financial)
-
- 25% improvement in high-risk application detection (QuickLoan Financial)
-
- 20% increase in annual returns (CapitalGains Investments)
-
- 30% improvement in risk prediction accuracy (GlobalTrust Insurance)
-
- 35% increase in portfolio performance metrics (EquityPlus Investment)
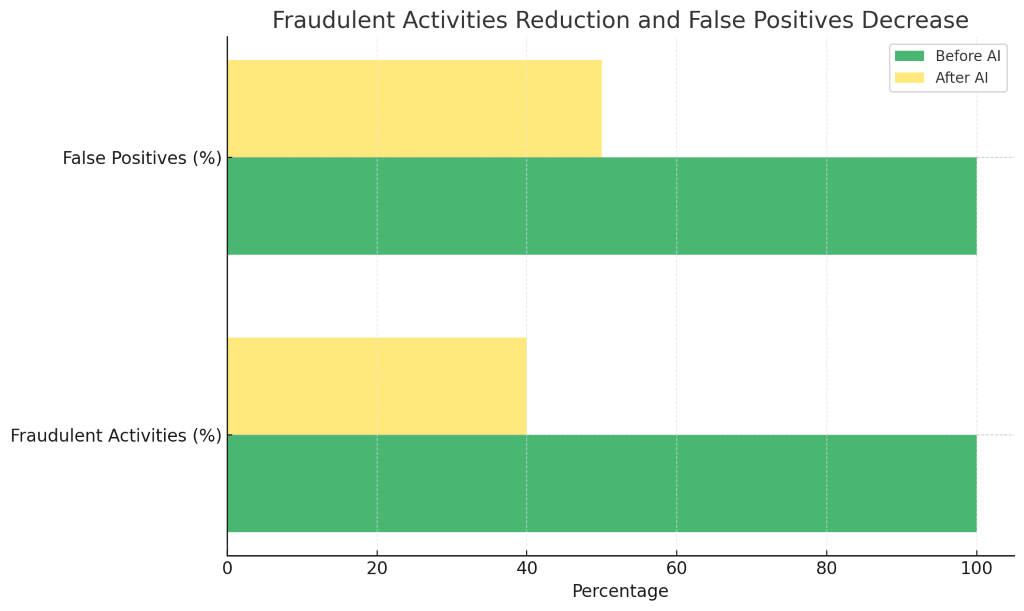
iii. Qualitative Benefits
AI enhances decision-making capabilities by providing data-driven insights. Tools like chatbots and AI-driven platforms improve customer satisfaction by offering immediate support and personalized services. Additionally, AI enables financial professionals to focus on strategic initiatives, such as risk management and investment planning.
iv. Long-term Benefits
Long-term benefits of AI in finance include continuous improvement in efficiency, enhanced customer experience, and better decision-making capabilities. AI’s ability to learn from data ensures that financial practices evolve with changing market conditions, fostering innovation and strategic foresight.
Cautions
i. Data Quality and Integration
Ensuring high data quality and seamless integration across systems is a primary challenge in AI adoption. Accurate data is crucial for AI tools to function effectively, and organizations must invest in robust data management strategies.
ii. Resistance to Change
AI adoption can face resistance from financial professionals accustomed to traditional processes. Overcoming this resistance requires effective change management strategies, including training programs and clear communication about the benefits of AI.
iii. Skill Development and Expertise
Effective use of AI in finance necessitates a skilled workforce capable of leveraging AI tools. Organizations need to invest in training and development programs to equip financial professionals with the necessary skills and knowledge.
iv. Ethical and Regulatory Considerations
AI deployment in finance must adhere to ethical standards and regulatory requirements. Organizations need to address potential biases in AI algorithms, ensure data privacy, and maintain transparency in AI-driven decisions.
v. Cost and Resource Allocation
Implementing AI in finance requires significant investment in technology, infrastructure, and training. Organizations must carefully evaluate the cost-benefit ratio and prioritize areas where AI can deliver the most substantial impact.
Conclusion
i. Summary of Key Findings
AI is revolutionizing the financial sector by automating processes, enhancing efficiency, and improving customer satisfaction. Institutions like FinSecure Bank, QuickLoan Financial, CapitalGains Investments, GlobalTrust Insurance, and EquityPlus Investment have successfully integrated AI into their operations, achieving significant time and cost savings.
ii. Overall Impact of AI on Financial Services
AI has fundamentally transformed financial roles, enabling professionals to focus on strategic activities and improving overall efficiency. The integration of AI tools fosters a more proactive and data-driven approach to finance, enhancing customer experience and organizational effectiveness.
iii. Recommendations for Financial Institutions Considering AI Adoption
For organizations considering AI adoption in finance, the following recommendations can help ensure successful implementation:
-
- Invest in Data Quality and Integration: Ensure high-quality, comprehensive data and seamless integration across systems.
-
- Foster a Positive Mindset: Emphasize the augmentative role of AI and provide training programs to help financial teams embrace the technology.
-
- Develop Necessary Skills: Invest in upskilling initiatives and ongoing education to equip financial professionals with AI expertise.
-
- Ensure Ethical and Regulatory Compliance: Establish governance frameworks to monitor AI activities, address biases, safeguard data privacy, and maintain transparency.
-
- Strategic Resource Allocation: Evaluate the cost-benefit ratio and prioritize areas where AI can deliver the most significant impact, starting with pilot projects and phased implementation